RAG Sample - 4. Build Embeddings and Insert to DB
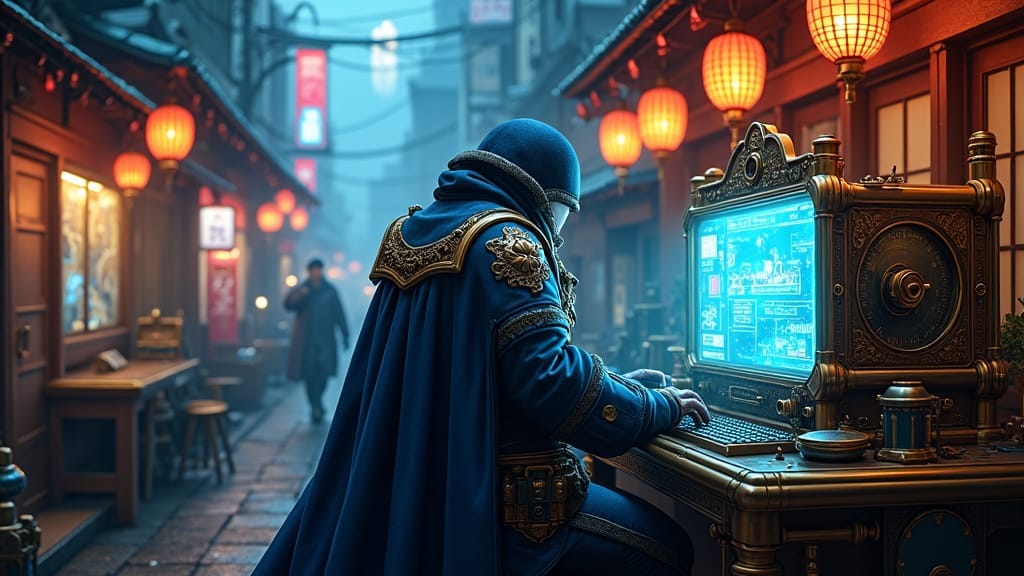
Building embeddings
I followed two sources for embeddings:
- Building an Agentic RAG locally with Milvus, Ollama and LangGraph and the associated code langgraph-rag-agent-local.ipynb
- The "Build RAG with Milvus | Milvus Documentation" tutorial
This is where I had my first surprise: Building embeddings is not necessarily a feature of the vector DB, and requires an embedding model. Fortunately, Ollama provides such models, and langchain has python bindings.
The first step is to create a OllamaEmbeddings
object:
ollama_emb = OllamaEmbeddings(model="mxbai-embed-large:latest", base_url="http://10.10.0.10:11434")
I used mxbai
because it's larger ™, but I've seen other tutorials that use nomic
just as well. mxbai
generates embeddings with a size of 1024 (and you need it for the schema). I did a test with llama3
, and it generated a 4096 item-long embedding. I guess a TODO is to see if it's worth using larger models for this stuff..
Once the embedding object has been created, we can embed documents (note a document is a bunch of text -- chunk):
ollama_emb.embed_documents(chunks)
My code looks like this:
from langchain_community.embeddings import OllamaEmbeddings
ollama_emb = OllamaEmbeddings(model="mxbai-embed-large:latest", base_url="http://10.0.0.35:11434")
def emb_text(text):
return (
ollama_emb.embed_documents([text])[0]
)
def emb_chunks(chunks):
return (
ollama_emb.embed_documents(chunks)
)
if __name__ == "__main__":
test_embedding = emb_text("This is a test, from other texts")
embedding_dim = len(test_embedding)
print(test_embedding)
print(embedding_dim)
It has the following characteristics:
emb_text
generates embeddings for a single stringemb_chunks
generates embeddings for an array of strings, one for each string- the embedding model is hardcoded, but it'll probably end up in a
dotenv
file - The main tries to embed a simple string and prints the embeddings vector and its length. As noted above,
mxbai
generates 1024-wide vectors.
Insert into the database
My current code looks like this:
uri = "http://10.10.0.10:19350"
collection_name = "my_data"
client = MilvusClient(uri=uri)
data = generate_embeddings(doc)
client.insert(collection_name, data)
connections.connect(client=client)
utility.wait_for_index_building_complete(
collection_name=collection_name,
index_name="_embeddings",
)
print(client.describe_collection(collection_name))
It:
- Builds a
MilvusClient
object - Generates the embeddings for the sample document
- Inserts the data into the collection
- Waits for the index to be built
- Prints collection statistics
Stay tuned for more!