RAG Sample - 1. Select a Vector DB
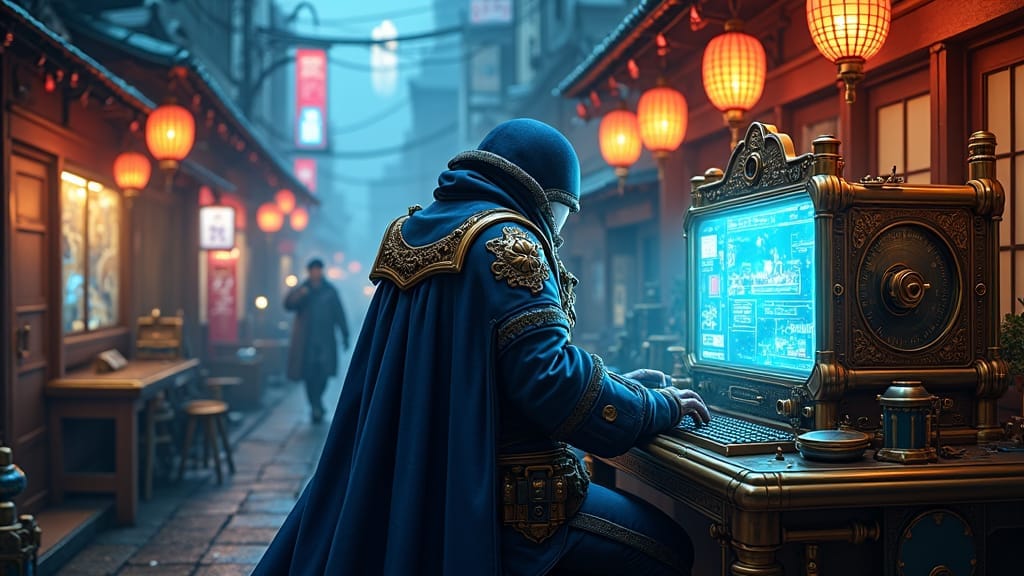
Create the RAG
Initially, I thought that RAG is a simple matter:
- Set up the vector DB
- Create and add the vectors to the DB
- Do a query in the DB with the prompt
- Get the results from the DB
- Feed the results and the prompt into the LLM
I'm finding out this is not so simple... I mean some of the steps are correct, but sometimes there are a lot of details an options, and you don't know what's best. So the stuff below are my attempts to build a RAG system.
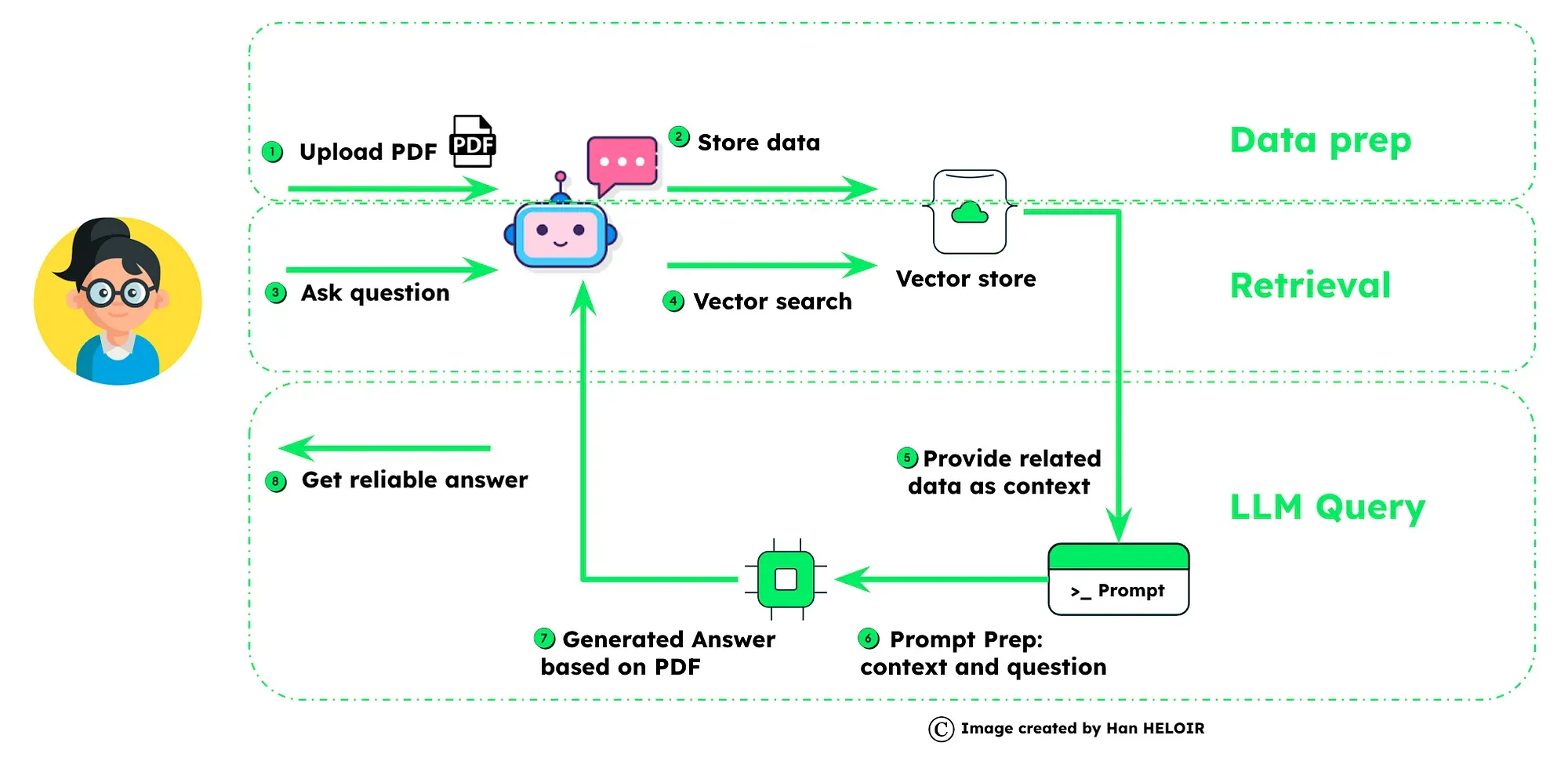
My use case
My use case is I think very common:
- I have Ollama with llama3 as reference model
- I have a bunch of documents that I would need to ask questions against, because Ollama doesn't know the summary of a paper my wife wrote 10 years ago.
My approach is to add a few documents/text data to the vector DB, play around with RAG and define the "best" variant for me. I will do it locally first (a bunch of scripts) and then attempt to do fancy stuff like a web interface, agents, a docker image.
Selecting a vector DB
I've looked for vector DBs for #RAG, and I've found several articles on the internet (I'm sure there are many more):
- The 5 Best Vector Databases | A List With Examples | DataCamp
- Implementing the pgvector extension for a PostgreSQL database | by Johannes Johansson | Medium
- Building LLM Applications With Vector Databases
I've narrowed it down to a handful:
- Chroma DB
- Weaviate
- Milvus
First I tried with Chroma, but it seemed to me it's using a sqlite backend. Then, I tried Weaviate, but the configuration wizard for Docker offered a bunch of options I'm not familiar with. Lastly, I've tried Milvus, which seems to have a plug-and-play docker-compose file.
Launching Milvus
I've downloaded the docker-compose file from here. At the moment of writing, it looks like this:
version: '3.5'
services:
etcd:
container_name: milvus-etcd
image: quay.io/coreos/etcd:v3.5.5
environment:
- ETCD_AUTO_COMPACTION_MODE=revision
- ETCD_AUTO_COMPACTION_RETENTION=1000
- ETCD_QUOTA_BACKEND_BYTES=4294967296
- ETCD_SNAPSHOT_COUNT=50000
volumes:
- ${DOCKER_VOLUME_DIRECTORY:-.}/volumes/etcd:/etcd
command: etcd -advertise-client-urls=http://127.0.0.1:2379 -listen-client-urls http://0.0.0.0:2379 --data-dir /etcd
healthcheck:
test: ["CMD", "etcdctl", "endpoint", "health"]
interval: 30s
timeout: 20s
retries: 3
minio:
container_name: milvus-minio
image: minio/minio:RELEASE.2023-03-20T20-16-18Z
environment:
MINIO_ACCESS_KEY: minioadmin
MINIO_SECRET_KEY: minioadmin
ports:
- "9001:9001"
- "9000:9000"
volumes:
- ${DOCKER_VOLUME_DIRECTORY:-.}/volumes/minio:/minio_data
command: minio server /minio_data --console-address ":9001"
healthcheck:
test: ["CMD", "curl", "-f", "http://localhost:9000/minio/health/live"]
interval: 30s
timeout: 20s
retries: 3
standalone:
container_name: milvus-standalone
image: milvusdb/milvus:v2.4.6
command: ["milvus", "run", "standalone"]
security_opt:
- seccomp:unconfined
environment:
ETCD_ENDPOINTS: etcd:2379
MINIO_ADDRESS: minio:9000
volumes:
- ${DOCKER_VOLUME_DIRECTORY:-.}/volumes/milvus:/var/lib/milvus
healthcheck:
test: ["CMD", "curl", "-f", "http://localhost:9091/healthz"]
interval: 30s
start_period: 90s
timeout: 20s
retries: 3
ports:
- "19530:19530"
- "9091:9091"
depends_on:
- "etcd"
- "minio"
networks:
default:
name: milvus
It has three components:
etcd
minio
- for block storagemilvus
- the vector DB
On launch, the vector DB will be available at http://localhost:19530
I've also launched attu to have a GUI for Milvus via docker:
docker run -p 8000:3000 -d -e MILVUS_URL=10.10.0.1019530 zilliz/attu:v2.
Now I have a pretty picture:
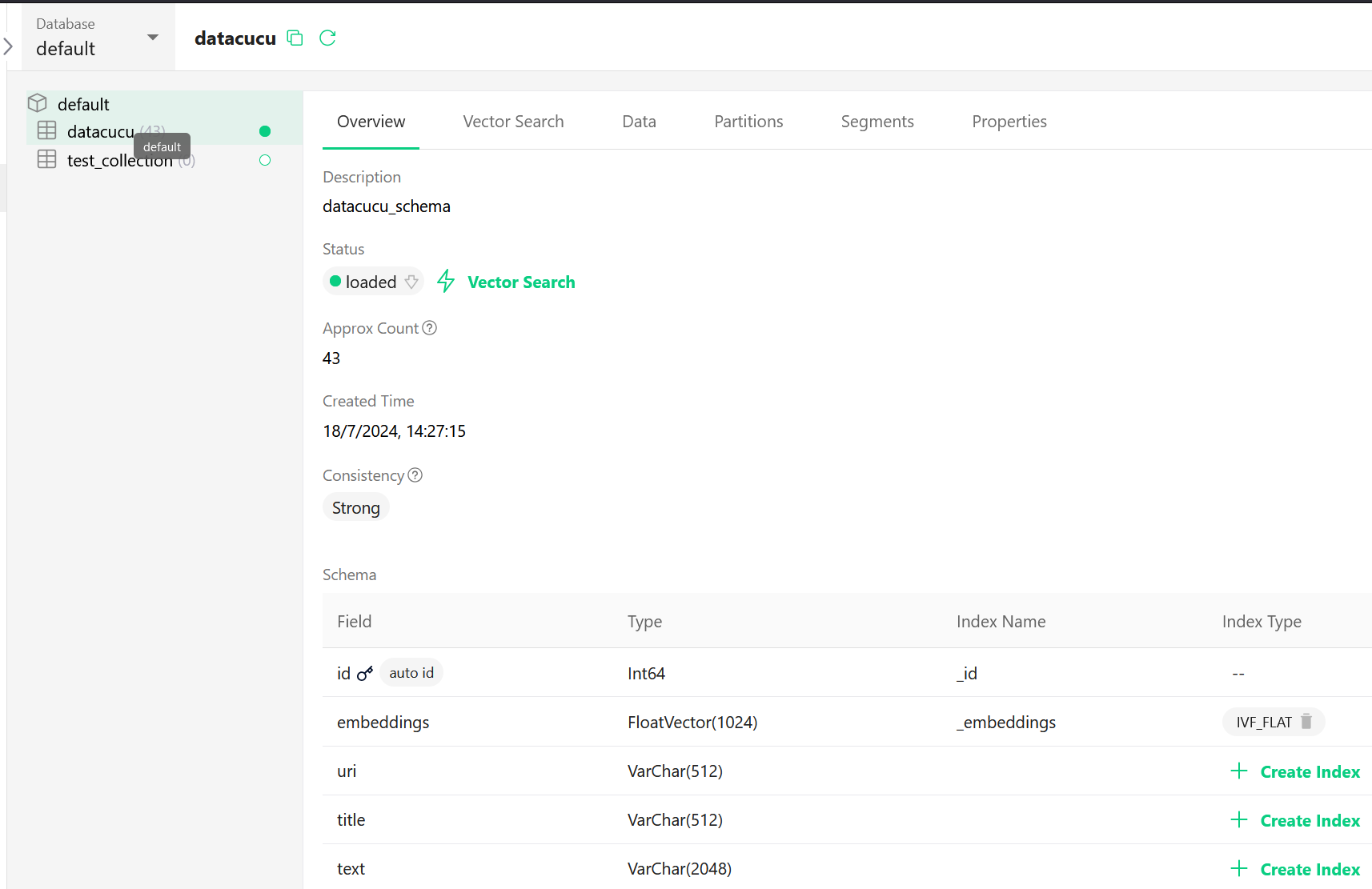
Is it useful? I'm not sure at this point and will come back later with a verdict, but sure it's pretty :)