Save Pandas DataFrame as Django Model
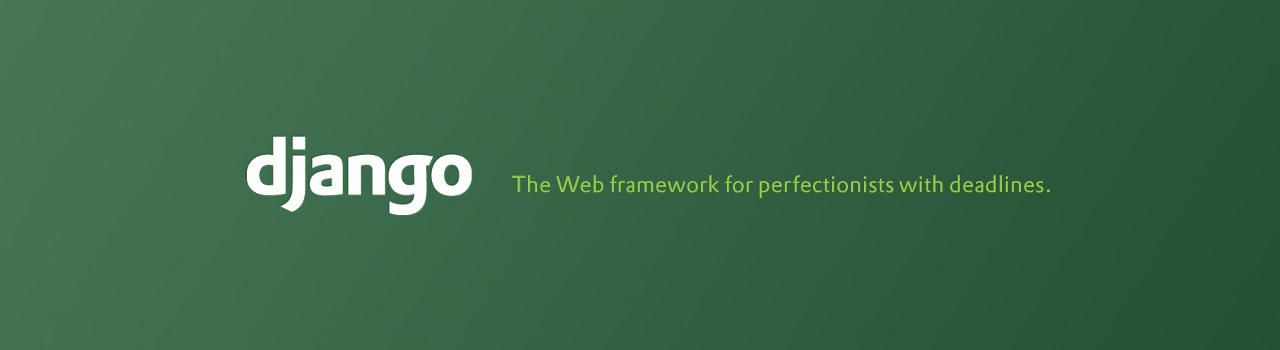
TL;DR: use bulk_upload
coupled with a comprehension to speed up loading.
Initially, I started to convert the data frame to a Model
object row by row and save it. While easy, it's also arguably the slowest method to load records, because the save()
call performs a commit
. Then I looked up how to save pandas data frames and I found out quite a few ways...
Manual commit
The first step would be to tweak the transaction to manually commit like described here:
from django.db import transaction
@transaction.commit_manually
def save(df):
...
for item in df.to_dict('records'):
entry = Entry(**item)
entry.save()
transaction.commit()
SQL
Other solution would be to use raw SQL. Here, you need to replicate the table fields by tweaking the data frame.
from django.conf import settings
user = settings.DATABASES['default']['USER']
password = settings.DATABASES['default']['PASSWORD']
database_name = settings.DATABASES['default']['NAME']
database_url = 'postgresql://{user}:{password}@localhost:5432/{database_name}'.format(
user=user,
password=password,
database_name=database_name,
)
engine = create_engine(database_url, echo=False)
df.to_sql(model._meta.db_table, con=engine)
Where:
model._meta.db_table
is the table where we want to savedatabase_url
is a sql_alchemy database URL (above configured for PostgreSQL)
This option has a few drawbacks:
- The URL needs to be manually created. If you have different DB engines for dev/test, you'll need to cater for that
- You'll need to add a
pk
column with incremental values - The code needs to be changed for each migration. Ouch!
Using bulk_create()
More recent versions of django have added bulk_create()
where you can pass an array of model objects and they get created at once. The code would be something like:
Model.objects.bulk_create(
Model(**vals) for vals in df.to_dict('records')
)
The operation works like this:
- Convert the dataframe in a list of dicts
- Create a
Model
object for each item in the list - Perform the
bulk_create()
on the list
This approach has some disadvantages too:
- It creates objects (not updates) so you can end up with duplicates if you're not careful
- You need to make sure the dict generated by a row can be used to create the model object
- It's slower than the SQL method above
- You might end up with lots of memory allocated if the dataframe is very large
I find the above disadvantages are minor compared to the simplicity of the execution.
Adapting DataFrame to Match
My approach to making sure the dict generated from a dataframe's row can be used to create a Model
object is:
-
Note the model's fields which are not nullable
-
Change the existing column names to match the field names
-
Pay attention and convert timestamp values to
datetime
values:df['timestamp'] = pd.to_datetime(df['timestamp'], unit='s')
Create any missing columns